Top 7 Challenges in Artificial Intelligence in 2023
Have you heard of Neuralink? It’s a young start-up company that Elon Musk helped co-found, and it’s working to seriously integrate artificial intelligence with the human body. They have created a chip that can be implanted into the brain and is made up of 96 thin polymer threads, each of which contains 32 electrodes.
Although I understand what you’re thinking, this is not serious science fiction. You can connect your brain with common electronic devices without even touching them by using this device, which is happening in the real world.
Here are some important questions to consider: Are we ready for this kind of technology? Will it really be that useful? How would it affect our lives in the future? Let’s explore the difficulties with AI.
Artificial intelligence has had a startling effect on both the economy and human lives. By 2030, artificial intelligence will have contributed $15.7 trillion to the global economy. That is roughly equivalent to the current economic output of China and India, to put it in perspective.
The number of AI start-ups has dramatically increased since 2000, with many businesses estimating that using AI can increase business productivity by up to 40%. AI can be used for a variety of tasks, such as tracking asteroids and other cosmic bodies in space, predicting diseases here on Earth, investigating fresh and creative methods to stop terrorism, and creating industrial designs.
Top common Challenges in AI:
1. Computing Power: The amount of energy used by these power-hungry algorithms is one factor that keeps most developers away. Machine Learning and Deep Learning are stepping stones to this AI and require an increasing number of cores and GPUs to function efficiently. There are several domains where we have ideas and knowledge to implement deep learning frameworks such as asteroid tracking, healthcare implementation, cosmic body tracking, and many more.
They need the computing power of a supercomputer and yes, supercomputers don’t come cheap. While the availability of cloud computing and parallel processing systems allows developers to work more effectively on AI systems, they come at a price. Not everyone can afford this with an increase in the influx of unprecedented amounts of data and rapidly increasing complex algorithms.
2. Lack of trust: A major concern for AI is the unknown nature of how deep learning models predict the output. How a specific set of inputs can provide a solution to different kinds of problems is difficult for the layman to understand. Many people in the world don’t even know the utility or existence of artificial intelligence and how it is integrated into the everyday objects they interact with, such as smartphones, smart TVs, banks and even cars (at some level of automation).
3. Limited Knowledge: Although there are many places in the market where we can use artificial intelligence as a better alternative to traditional systems. The real problem is the knowledge of artificial intelligence. Aside from tech enthusiasts, students, and researchers, there are only a limited number of people who are aware of the potential of AI.
For example, many SMEs (small and medium-sized enterprises) plan their work or learn innovative ways to increase their production, manage their resources, sell and manage products online, learn and understand the behaviour of consumers and respond efficiently and effectively to the market. can react. They are also unfamiliar with service providers like Google Cloud, Amazon Web Services, and others in the tech industry.
4. Human level: This is one of the major AI challenges that has kept researchers busy with AI services in enterprises and startups. These companies may boast over 90% accuracy, but humans can do better in all of these scenarios. For example, let our model predict whether the image is of a dog or a cat. Humans can predict the right output nearly every time, with an astonishing accuracy of over 99%.
For a deep learning model to provide comparable performance, it requires unprecedented tuning, hyperparameter tuning, a large dataset, and a well-defined and accurate algorithm, along with robust computational power, ongoing data education of the train and test on the test data. It sounds like a lot of work, and it’s actually a hundred times harder than it sounds.
One way to avoid all the hard work is to simply use a service provider that can train specific deep learning models using pre-trained models. They’ve been trained on millions of images and are fine-tuned for maximum accuracy, but the real problem is that they keep showing errors and would really struggle to achieve human-level performance.
5. Confidentiality and Data Security: The most important factor on which all deep learning and machine learning models rely is the availability of data and resources to train them. Yes, we have data, but since this data is generated by millions of users around the world, there is a possibility that this data could be used for illegal purposes.
Suppose a healthcare provider provides services to 1 million people in a city, and a cyberattack causes the personal information of all 1 million users to fall into everyone’s hands on the dark web. This data includes data on diseases, health conditions, medical history and much more. To make matters worse, we are now dealing with planet-size data. With so much information coming in from all directions, there would definitely be some cases of data breaches.
Some companies have already started innovative work to circumvent these barriers. It trains the data on smart devices and then isn’t sent back to the servers, only the trained model is sent back to the organization.
6. Problem of Prejudice: The good or bad nature of an AI system really depends on how much data it has been trained on. Therefore, the ability to get good data is the key to good AI systems in the future. But in reality, the daily data that organizations collect is scarce and meaningless.
They are biased and only somewhat define the nature and specifics of a small number of people with common interests based on religion, ethnicity, gender, community, and other racial biases. Real change can only be brought about by defining algorithms that can effectively track these issues.
7. Scarcity of Data: While big companies like Google, Facebook and Apple are accused of unethical use of generated user data, different countries like India are enforcing strict IT rules to restrict the flow of data. These companies are therefore now faced with the problem of using local data to develop applications for the whole world, which would lead to bias.
Data is a very important aspect of AI and labelled data is used to train, learn and make predictions about machines. Some companies are trying to develop new methods and focus on creating AI models that can provide accurate results despite the scarcity of data. With distorted information, the whole system can become flawed.
Related Posts
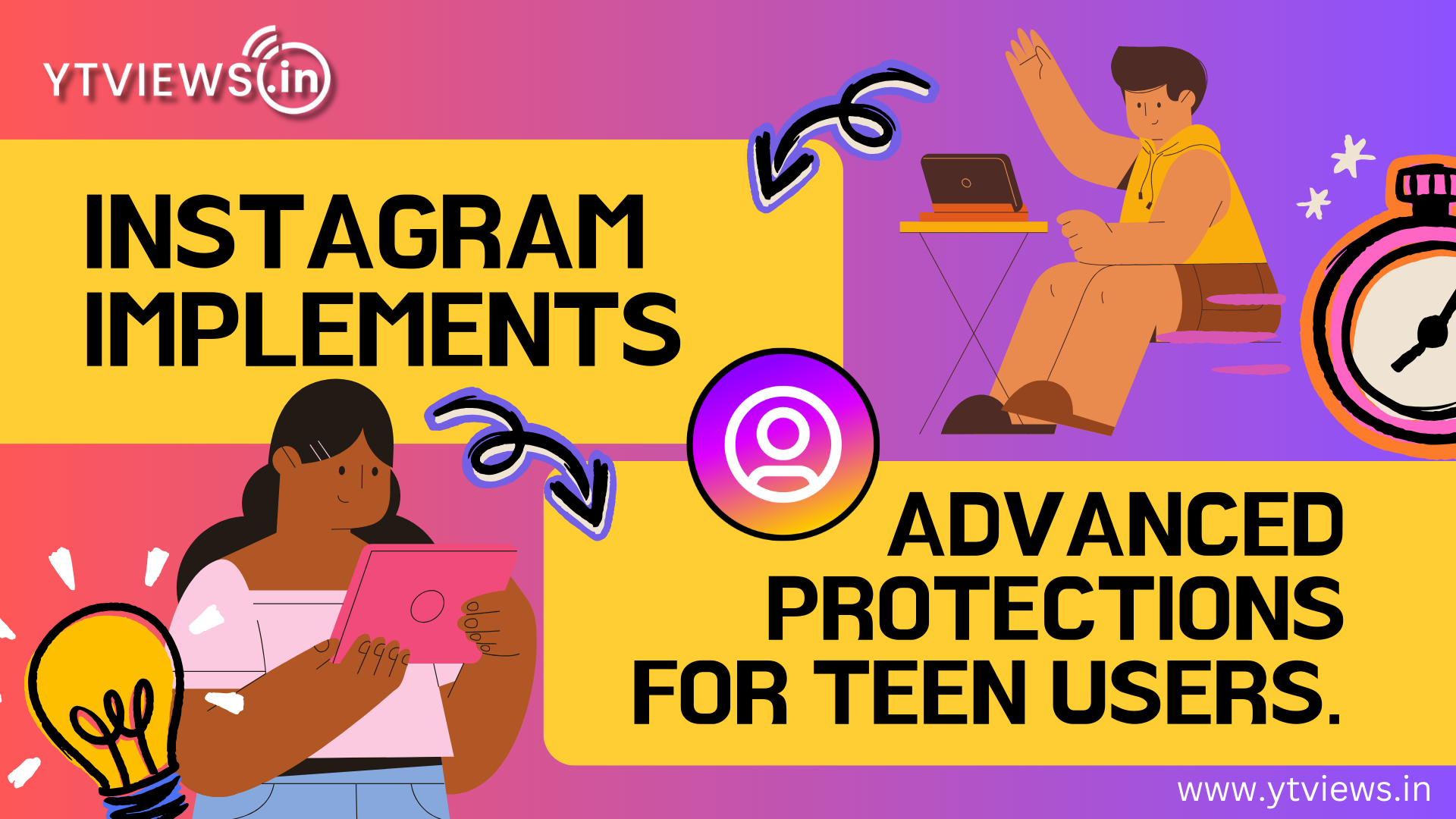
Instagram Implements Advanced Protections for Teen Users.
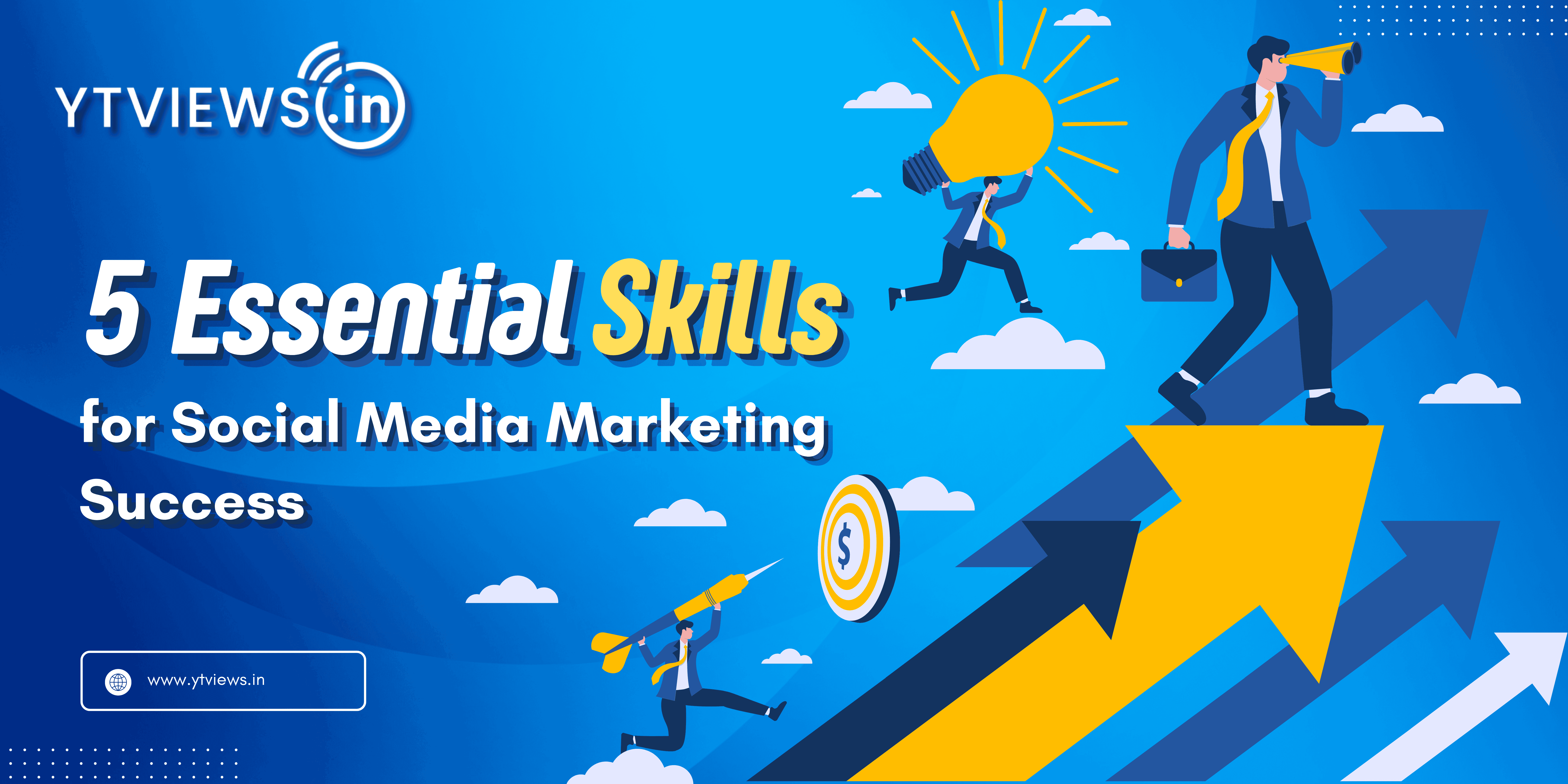
5 Skills to Become a Successful Social Media Marketer
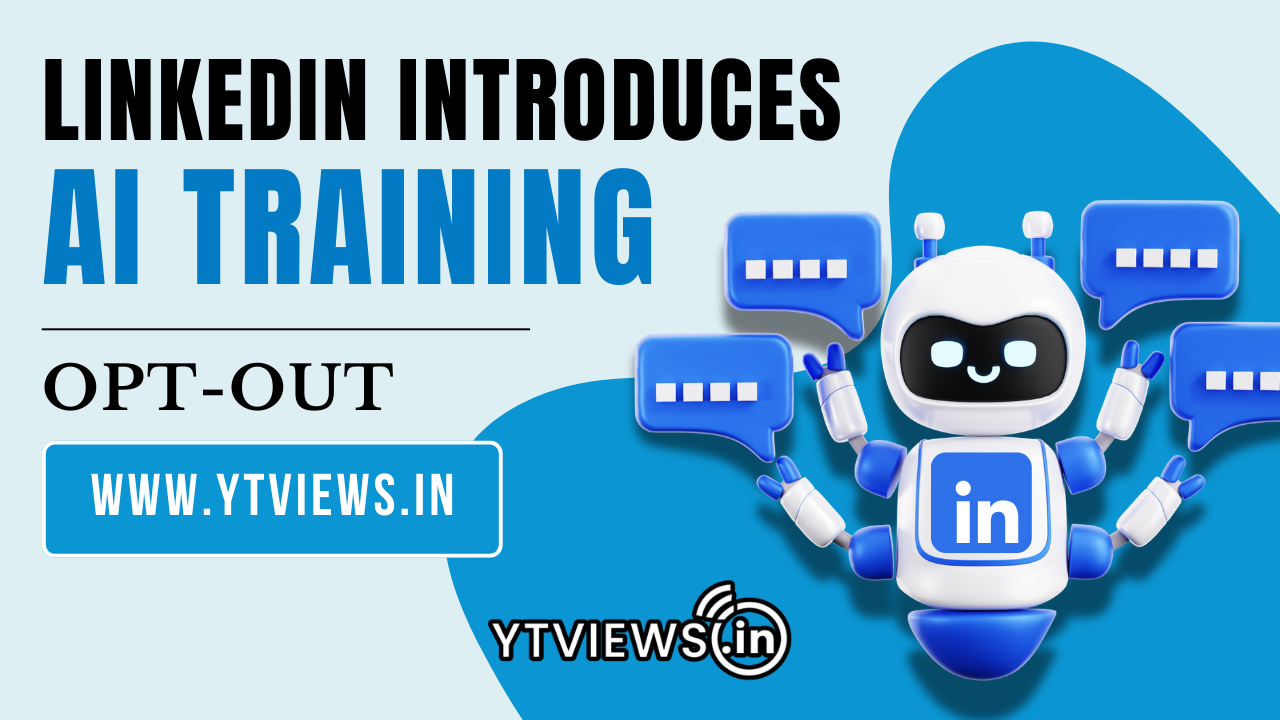
LinkedIn Adds AI Training Opt-out Option
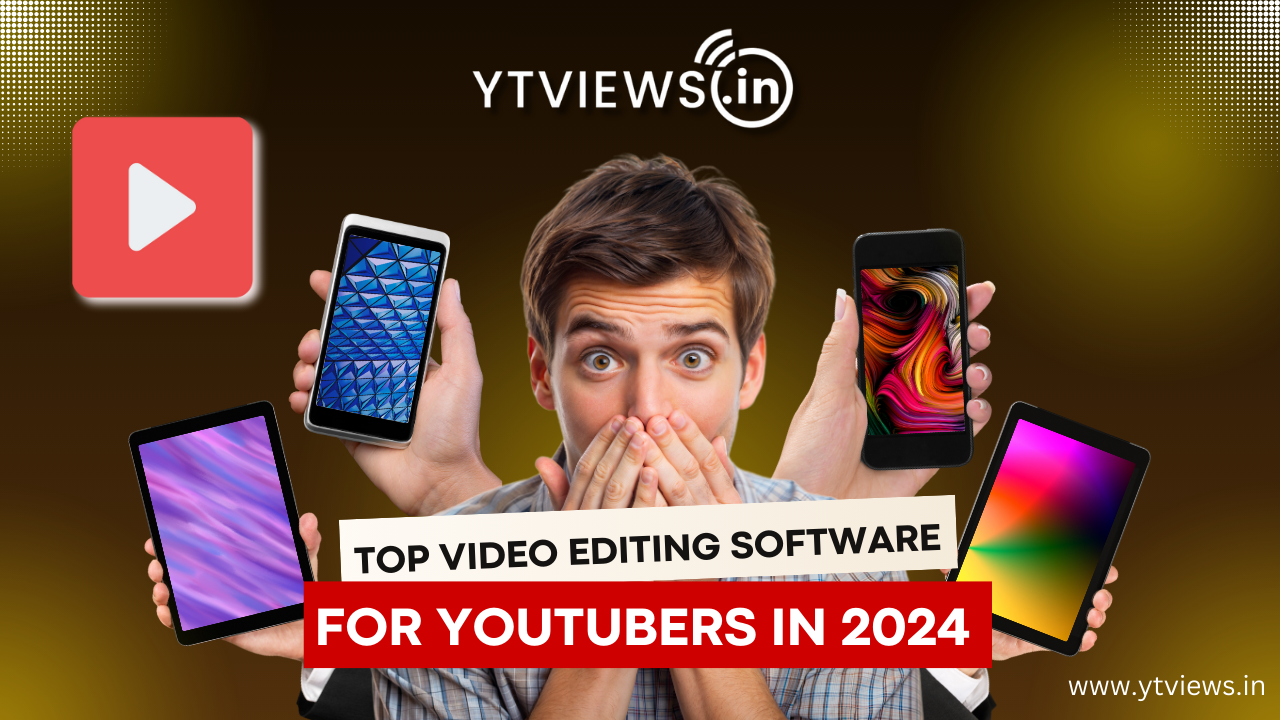
What Video Editing Software Do Youtubers Use in 2024?
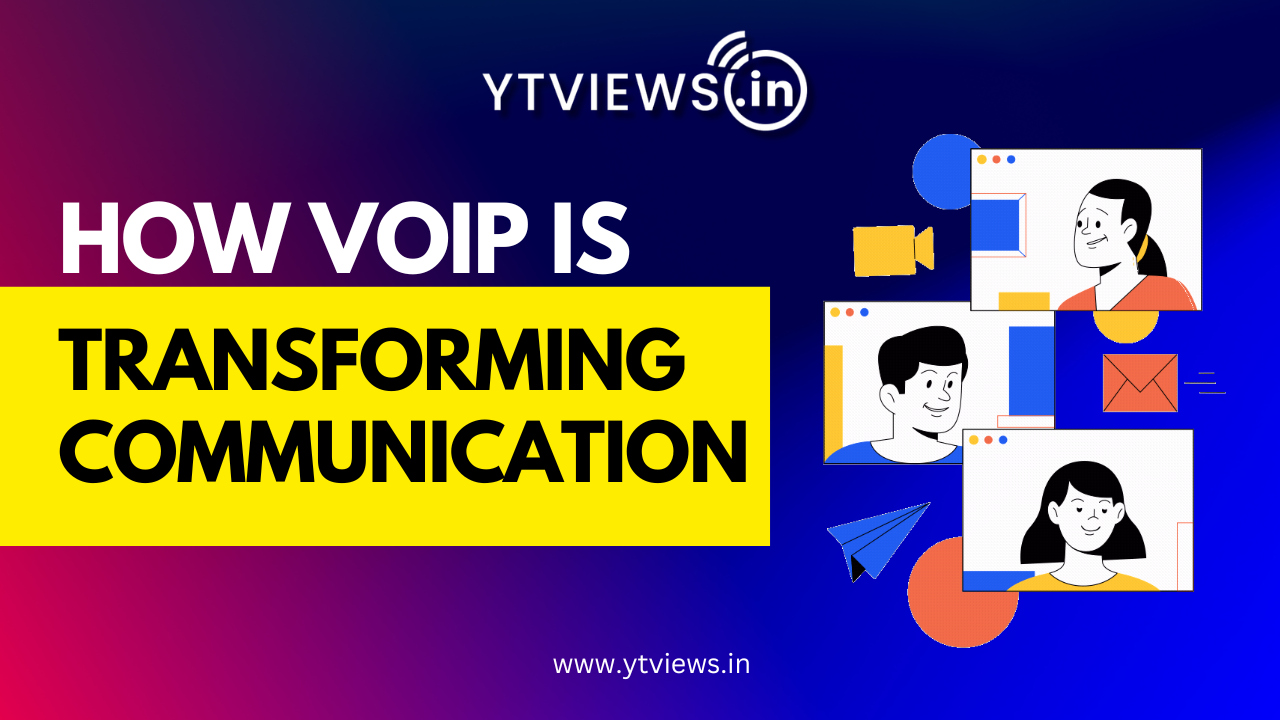
How VoIP Services are changing the Way We Make Calls
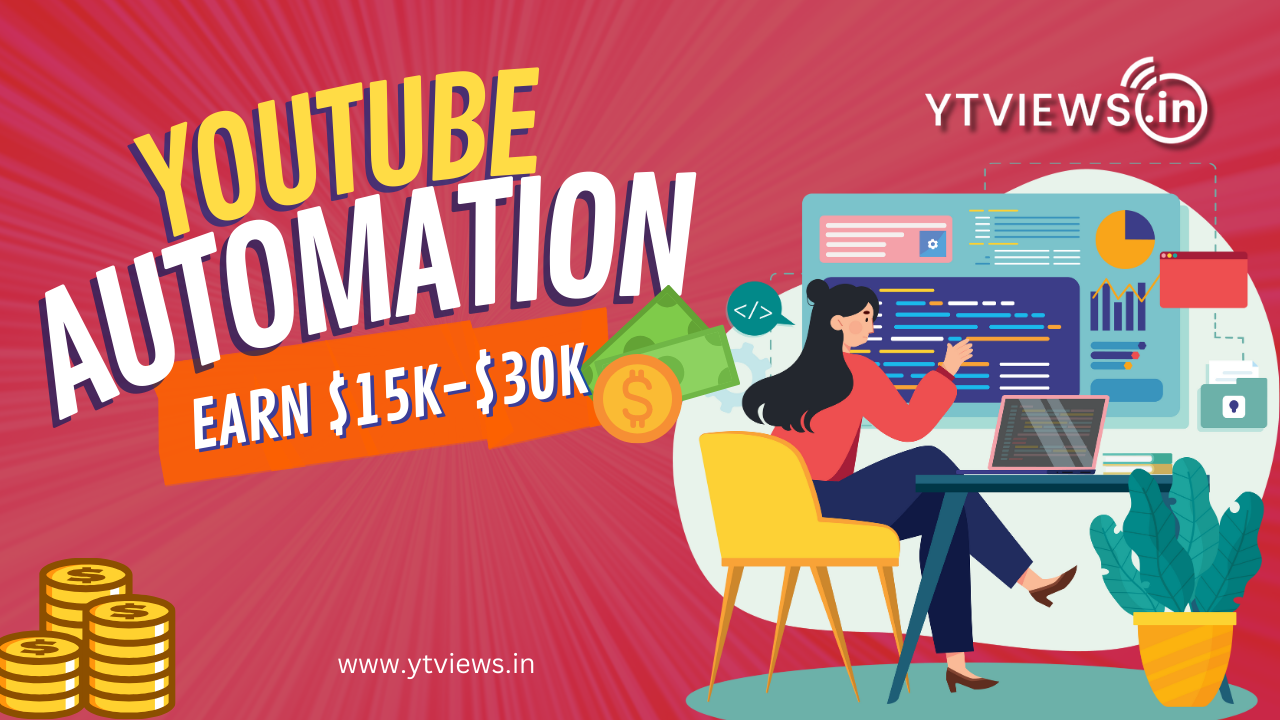